Will it scale? Webinar addresses pitfalls and opportunities around intervention scaling
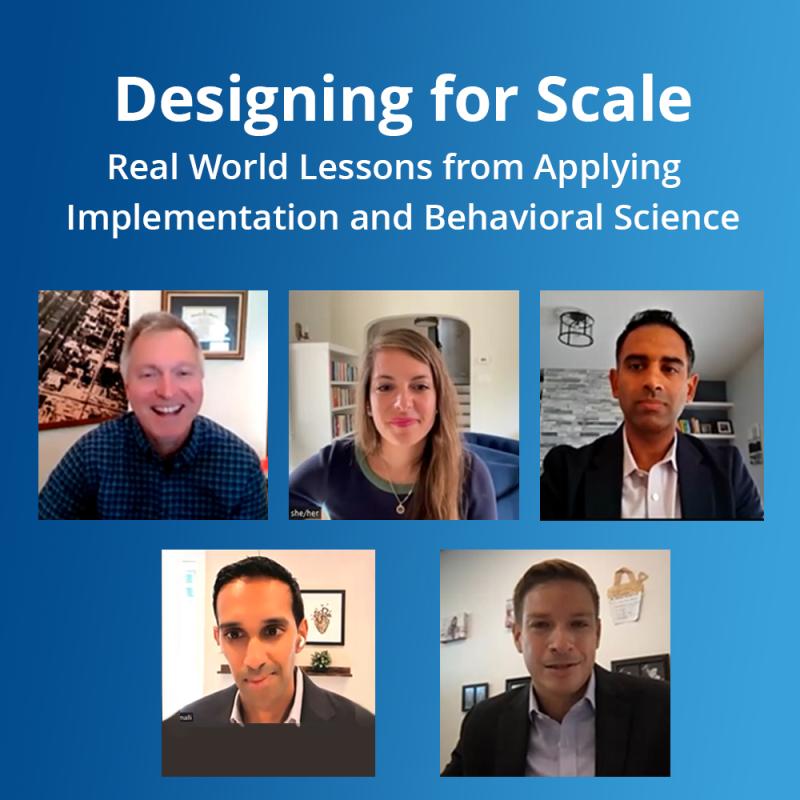
In health care and many other fields, initiatives may lead to desired outcomes in a limited population or area but often fizzle when rolled out more broadly. What causes failure at scale, and how can teams adjust program design and implementation to achieve scaling success? Experts in behavioral economics and implementation science came together virtually on October 14 to share their perspectives on scaling in an event hosted by the Penn Medicine Nudge Unit.
Headlining the program was John A. List, PhD, Kenneth C. Griffin Distinguished Service Professor in Economics at the University of Chicago, chief economist at Walmart, and author of The Voltage Effect: How to Make Good Ideas Great and Great Ideas Scale. After a keynote by List, three past Nudge Unit leaders joined present Nudge director M. Kit Delgado, MD, MS, for a panel discussion:
- Srinath Adusumalli, MD, MSHP, MBMI, Senior Medical Director of Enterprise Virtual Care at CVS Health,
- Rinad Beidas, PhD, Chair and Ralph Seal Paffenbarger Professor of Medical Social Sciences at Northwestern University Feinberg School of Medicine, and
- Mitesh Patel, MD, MBA, National Vice President for Clinical Transformation and National Lead for Behavioral Insights at Ascension.
Checking the “vital signs” of scalability
“Ideas have five vital signs that make them click or cause them to be predictably unscalable,” List said. He described three of those signs, distilled from his field experiments and scholarship, and illustrated with examples from the policy, education, and technology sectors.
False positives can yield a mistaken belief that a project is scalable. False positives come from not only statistical error but also human error – in generating and interpreting data – and fraud. “All of these combine to lead to a much higher false positive rate than what we advertise as 5 percent. And, in this case, the importance of replication becomes very clear,” said List.
Knowing the audience means considering whether an idea or results apply to people beyond those in the trial group. As an example, List cited a “smart” technology that was developed to help households save energy but failed because consumers used it differently from the way the engineers intended.
“In health and health care, we see this a lot, in that people think a new app or technology solution is going to solve a lot of things,” said Patel, summarizing a JAMA opinion piece he coauthored about wearable devices and health behavior change. “What we find when we implement it is that those things are really facilitators, but we have to think about how we’re going to drive behavior change with the people that are using our technology, whether or not they use it at all, and if they use it in the ways that they’re supposed to. You need interventions that do both: You need to implement technology that hopefully reduces your work or automates something. And you also need processes in place that drive behavior change amongst the people that are using the technology to enable the ultimate outcome.”
Scalability also depends on whether a program’s success relies on the chef or the ingredients. If the chef is the key, said List, “That’s setting yourself up for failure because unique humans typically do not scale. If your initial success is based on some ingredients, and those ingredients are available at scale, now you have a shot, as long as you execute.”
List advocated going beyond efficacy (A/B) tests, recommending that researchers set up an “option C” to test a program “with all of the warts and constraints” it would face at scale. These might include regulatory or input constraints. Understanding the mediation path leading to an outcome can also help teams determine whether a project can scale, said List.
Applying an implementation science lens
“There’s a lot that implementation science can bring to the field of behavioral economics and behavioral science, just like the other way around,” said Beidas, an international expert in the field of implementation science.
She described two key ideas in implementation science that relate to scaling. First, an emphasis on context: interventions should be designed with attention to the situations in which they will be delivered and in partnership with the people who will receive and deliver the interventions. Second, implementation science involves evidence-based practices for understanding context, deploying interventions, assessing results, and iterating.
Beidas added that, when designing interventions, teams should strive “to keep equity and care team wellness at the forefront, and to ensure that when we evaluate our approaches, those are things that we’re also evaluating.”
Opportunities in health informatics
“One thing that I commonly see is that we develop different clinical decision support tools or things to change the system to make it easier for clinicians to do the right thing,” Delgado noted. “But it’s hard to scale these across health systems.”
Picking up on this point, Adusumalli shared insights about the informatics landscape: specifically, electronic health records (EHRs), which have become a prominent tool in health care in recent years and have been used to deliver nudges like default orders, prescription defaults, and active choice prompts.
“Interoperability of health IT systems and EHRs is nowhere near a solved problem, but there has been meaningful progress,” said Adusumalli, listing examples such as health information exchanges, legislation that promotes data sharing, and common data models.
Adusumalli added that most health systems in the United States are using a limited set of EHRs, and commonalities among those EHRs offer an opportunity for sharing data and deploying interventions across sites. Separately, applications that function on common standards “can contain the nudge intervention itself and plug into different EHRs.”
Individual- versus system-framed interventions
Citing a 2022 paper that has sparked debate, Delgado invited the panel to discuss individual-level versus system-level framing for behavioral change interventions and the suggestion that individual-framed interventions are prone to losing replicability or impact at scale.
“It’s probably always going to be a ‘both-and’ answer,” said Beidas. “For too long, we’ve focused on individual-level behavior change because it’s easier to intervene at that level. But structural problems require structural solutions.”
One-size-fits-all approaches can be problematic, said Patel, but a promising direction for realizing change at scale is to blend system-level interventions with individualized points of connection.
List addressed the topic from the perspective of generalizing across people versus situations. “You’re going to have a better shot at changing behaviors in a big and deep way if you control the situation versus a representation of the people,” he said. “How can we change the representativeness of the situation and test that representativeness within a model to try to figure out how behaviors will change? I think that will unlock a lot of the deeper secrets about how to make change at scale.”